Read a netcdf file and make a contour plot of the data¶
In this example, we demonstrate: 1. How to read a netcdf file in Python using xarray
2. How to make a contour plot of the data
Data¶
We will read CMIP5 data for surface air temperature (tas) from the RCP8.5 scenario produced by the NCAR/CCSM4 model. For this example, we will read teh first ensemble member.
The data are located on the COLA severs in the following directory: /shared/cmip5/data/rcp45/atmos/mon/Amon/tas/NCAR.CCSM4/r1i1p1/
The filename is: tas_Amon_CCSM4_rcp45_r1i1p1_210101-229912.nc
Python import statements¶
You must first import the Python packages you wish to use. This is a common set of basic import statments you can start with.
[1]:
import numpy as np
import xarray as xr
import matplotlib.pyplot as plt
Set the path and filename
[2]:
path='/shared/cmip5/data/rcp45/atmos/mon/Amon/tas/NCAR.CCSM4/r1i1p1/'
fname='tas_Amon_CCSM4_rcp45_r1i1p1_200601-210012.nc'
Read the data using xarray
open_dataset
http://xarray.pydata.org/en/stable/generated/xarray.open_dataset.html
[3]:
ds=xr.open_dataset(path+fname)
When you read in data using xarray
, it creates an object called an xarray.Dataset
which consists of your data and all its metadata. If we print out our Dataset
which is called ds
, its similar to doing a ncdump -h
on a netcdf file. You can see all the dimensions, size, and attributes of the data in the file.
[4]:
ds
[4]:
<xarray.Dataset> Dimensions: (bnds: 2, lat: 192, lon: 288, time: 1140) Coordinates: * time (time) object 2006-01-16 12:00:00 ... 2100-12-16 12:00:00 * lat (lat) float64 -90.0 -89.06 -88.12 -87.17 ... 88.12 89.06 90.0 * lon (lon) float64 0.0 1.25 2.5 3.75 5.0 ... 355.0 356.2 357.5 358.8 height float64 ... Dimensions without coordinates: bnds Data variables: time_bnds (time, bnds) object ... lat_bnds (lat, bnds) float64 ... lon_bnds (lon, bnds) float64 ... tas (time, lat, lon) float32 ... Attributes: institution: NCAR (National Center for Atmospheric Resea... institute_id: NCAR experiment_id: rcp45 source: CCSM4 model_id: CCSM4 forcing: Sl GHG Vl SS Ds SA BC MD OC Oz AA parent_experiment_id: historical parent_experiment_rip: r1i1p1 branch_time: 2005.0 contact: cesm_data@ucar.edu references: Gent P. R., et.al. 2011: The Community Clim... initialization_method: 1 physics_version: 1 tracking_id: 0bf35136-b266-44d2-9078-f3081b83b6ad acknowledgements: The CESM project is supported by the Nation... cesm_casename: b40.rcp4_5.1deg.001 cesm_repotag: ccsm4_0_beta49 cesm_compset: BRCP45CN resolution: f09_g16 (0.9x1.25_gx1v6) forcing_note: Additional information on the external forc... processed_by: strandwg on mirage0 at 20111021 processing_code_information: Last Changed Rev: 428 Last Changed Date: 20... product: output experiment: RCP4.5 frequency: mon creation_date: 2011-10-21T21:56:22Z history: 2011-10-21T21:56:22Z CMOR rewrote data to c... Conventions: CF-1.4 project_id: CMIP5 table_id: Table Amon (26 July 2011) 976b7fd1d9e1be31d... title: CCSM4 model output prepared for CMIP5 RCP4.5 parent_experiment: historical modeling_realm: atmos realization: 1 cmor_version: 2.7.1
If you want to access just the surface air tempeature (tas) data itself, without all the gloal attributes, you can do that by supplying the name of the variable
[5]:
ds['tas']
[5]:
<xarray.DataArray 'tas' (time: 1140, lat: 192, lon: 288)> [63037440 values with dtype=float32] Coordinates: * time (time) object 2006-01-16 12:00:00 ... 2100-12-16 12:00:00 * lat (lat) float64 -90.0 -89.06 -88.12 -87.17 ... 87.17 88.12 89.06 90.0 * lon (lon) float64 0.0 1.25 2.5 3.75 5.0 ... 355.0 356.2 357.5 358.8 height float64 ... Attributes: standard_name: air_temperature long_name: Near-Surface Air Temperature units: K original_name: TREFHT comment: TREFHT no change cell_methods: time: mean (interval: 30 days) cell_measures: area: areacella history: 2011-10-21T21:56:22Z altered by CMOR: Treated scalar d... associated_files: baseURL: http://cmip-pcmdi.llnl.gov/CMIP5/dataLocation...
matplotlib
plt.contourf
function for a filed contour plot. It works very similar to Matlab plotting functions.[6]:
plt.contourf(ds['tas'][0,:,:])
plt.colorbar()
[6]:
<matplotlib.colorbar.Colorbar at 0x7f95fc109c88>
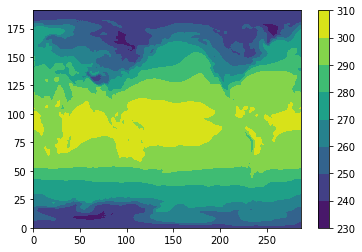
This is a very simple plot, but it looks like we have global temperature data. More details on how to plot maps, make nice lables, and colors, can be found in other examples.
[ ]: