Read a file from an remote OPeNDAP server and make a contour plot of the data¶
In this example, we demonstrate: 1. How to read a netcdf file in Python using xarray
from a remote OPeNDAP server 2. How to make a contour plot of the data
Data¶
We will read data from the North American Multi-model Ensemble (NMME) database. Specifially, we will read the sea surface temperature (SST) data hindcast data for the COLA-RSMAS-CCSM4 model.
The NMME public database is hosted on the International Research Institute for Climate and Society (IRI) data server http://iridl.ldeo.columbia.edu/SOURCES/.Models/.NMME/
Python import statements¶
You must first import the Python packages you wish to use. This is a common set of basic import statments you can start with.
[7]:
import numpy as np
import xarray as xr
import matplotlib.pyplot as plt
Set the path and filename
[8]:
url = 'http://iridl.ldeo.columbia.edu/SOURCES/.Models/.NMME/.COLA-RSMAS-CCSM4/.MONTHLY/.sst/dods'
Read the data using xarray
open_dataset
http://xarray.pydata.org/en/stable/generated/xarray.open_dataset.html
[9]:
ds =xr.open_dataset(url, decode_times=False)
When you read in data using xarray
, it creates an object called an xarray.Dataset
which consists of your data and all its metadata. If we print out our Dataset
which is called ds
, its similar to doing a ncdump -h
on a netcdf file. You can see all the dimensions, size, and attributes of the data in the file.
[10]:
ds
[10]:
<xarray.Dataset> Dimensions: (L: 12, M: 10, S: 457, X: 360, Y: 181) Coordinates: * S (S) float32 264.0 265.0 266.0 267.0 ... 717.0 718.0 719.0 720.0 * X (X) float32 0.0 1.0 2.0 3.0 4.0 ... 355.0 356.0 357.0 358.0 359.0 * M (M) float32 1.0 2.0 3.0 4.0 5.0 6.0 7.0 8.0 9.0 10.0 * L (L) float32 0.5 1.5 2.5 3.5 4.5 5.5 6.5 7.5 8.5 9.5 10.5 11.5 * Y (Y) float32 -90.0 -89.0 -88.0 -87.0 -86.0 ... 87.0 88.0 89.0 90.0 Data variables: sst (S, L, M, Y, X) float32 ... Attributes: Conventions: IRIDL
If you want to access just the surface air tempeature (tas) data itself, without all the gloal attributes, you can do that by supplying the name of the variable
[11]:
ds['sst']
[11]:
<xarray.DataArray 'sst' (S: 457, L: 12, M: 10, Y: 181, X: 360)> [3573374400 values with dtype=float32] Coordinates: * S (S) float32 264.0 265.0 266.0 267.0 ... 717.0 718.0 719.0 720.0 * X (X) float32 0.0 1.0 2.0 3.0 4.0 ... 355.0 356.0 357.0 358.0 359.0 * M (M) float32 1.0 2.0 3.0 4.0 5.0 6.0 7.0 8.0 9.0 10.0 * L (L) float32 0.5 1.5 2.5 3.5 4.5 5.5 6.5 7.5 8.5 9.5 10.5 11.5 * Y (Y) float32 -90.0 -89.0 -88.0 -87.0 -86.0 ... 87.0 88.0 89.0 90.0 Attributes: defaultvalue: 720.0 pointwidth: 0 long_name: Sea Surface Temperature cell_methods: time: mean units: Celsius_scale spatial_op: Conservative remapping: 1st order: destarea: NCL: /homes/... lat: 89.5 standard_name: sea_surface_temperature expires: 1580517720
The NMME data dimensions correspond to the following: X=lon,L=lead,Y=lat,M=ensemble member, S=initialization time.
matplotlib
plt.contourf
function for a filed contour plot. It works very similar to Matlab plotting functions.[12]:
plt.contourf(ds['sst'][0,0,0,:,:])
plt.colorbar()
[12]:
<matplotlib.colorbar.Colorbar at 0x7f28e4d47438>
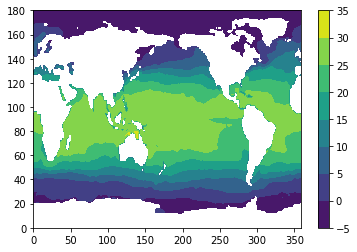
This is a very simple plot, but it looks like we have global temperature data. More details on how to plot maps, make nice lables, and colors, can be found in other examples.